Building the 2018 Ibrahim Index of African Governance: methodology explained
10 October, 2018
The 2018 Ibrahim Index of African Governance (IIAG) is the 12th iteration of this Index, and is the most comprehensive annual statistical assessment of the quality of governance in 54 African countries across the framework of the Index. So how is it built?
The journey of a data point
Collection and variables selection
The Mo Ibrahim Foundation is not a primary data provider: the data used to calculate the IIAG is exclusively provided by external sources. In the 2018 IIAG, data come from 35 international sources such as UN agencies, the World Bank and African Development Bank, but also data projects (e.g. Armed Conflict Location & Events Data Project) and surveys (e.g. Afrobarometer).
To ensure the Index is statistically robust and comparable between countries, the data are required to meet a number of criteria:
- be a suitable proxy for governance
- cover at least 33 African countries
- provide at least two years’ worth of data for these countries since 2008
- the latest data point can be no more than three years old.
Moreover, to differentiate between scores, numerical granularity is taken into consideration, with all the variables selected being on a four-point scale or more.
Data treatment
Outliers
Some variables might include values that lie outside from the mass of the rest of the distribution – called outliers. Including these extreme observations in the IIAG would bias variable scores and mean that after normalisation the range would be skewed, making comparisons between most of the country scores difficult. To prevent this, the raw data is analysed to determine whether any of the variables require treatment to address outliers.
To identify the outliers, a diagnostic is performed in the raw data for each variable collected. All data points that lie more than three trimmed standard deviations from the trimmed mean are replaced with: trimmed mean + (3.1 x trimmed standard deviations) if they are in the right-hand tail of the distribution; and trimmed mean – (3.1 x trimmed standard deviations) if they are in the left-hand tail. The trimmed moments are computed on the 95% central part of the distribution (i.e. removing those observations that are in the bottom 2.5% and top 2.5%).
In the 2018 IIAG, six variables where treated for outliers: Absence of Riots & Protests; Absence of Government Violence against Civilians; Absence of Violence against Civilians by Non-state Actors; Absence of Internally Displaced Persons; Absence of Refugees; and Absence of Malaria.
Missing data
As is frequent with data projects, the majority of the variables included in the IIAG have a degree of missing data, as not all sources publish data for all years and all countries. To ensure continuity and comparability between composite scores over time, it is necessary to estimate values for these years.
Missing data can be located in the interior of the available time series or at the exterior. For the former, the linear interpolation method is used – values are replaced with numbers incrementally higher or lower than the neighbouring data points. For the latter, the missing values are replaced using the closest data point from source (last value carried forward – LVCF – or first value carried backward – FVCB).
Normalisation
Data collected to compute the IIAG are diverse. At source, the variables collected are produced on different scales (e.g. number of refugees can be infinite, or robustness of banks can be bounded on a scale 1-7), and can also have different polarities – higher is better or higher is worse. In order for them to be meaningfully combined and compared, raw data are standardised before being included in the IIAG.
The Foundation employs a statistical process called min-max normalisation whereby all raw data are transformed to a scale of 0.0-100.0 (where a score of 100.0 is the best score a country can achieve). While this constitutes an order-preserving linear transformation of the data, a score of 100.0 after normalisation does not imply that a country’s score in raw data terms is perfect, but rather is the best score on the continent.
Aggregation and weighting
The IIAG uses linear, additive aggregation and weights each sub-component equally within its dimension (e.g. a country’s score in Overall Governance is arrived at by calculating an unweighted average of its four underlying categories – Safety & Rule of Law, Participation & Human Rights, Sustainable Economic Opportunity and Human Development).
While the weight of all four categories is equal in the Overall Governance composite score, sub-categories have different implicit weightings as a result of the structure of the IIAG. For example, while Human Development is composed of three sub-categories (Welfare, Education and Health), Safety & Rule of Law has four sub-categories (Rule of Law, Transparency & Accountability, Personal Safety, and National Security).
Clustering
A clustered indicator is composed of a number of underlying variables which capture the same dimension. An indicator can be clustered when data on the same narrow issue is available from multiple sources, in order to improve the accuracy of the indicator measurement and avoid over-representing the weight of each variable. For example, Fiscal Policy is measured by two different data sources included in the IIAG: the African Development Bank (AfDB) and the World Bank (WB).
The 2018 data will be released on 29 October.
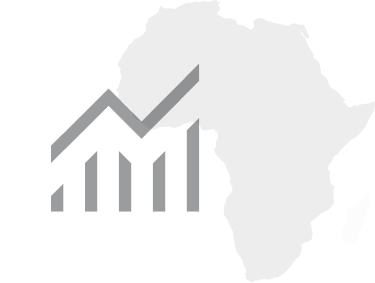